[1]:
#!/usr/bin/env python
Demo: trinidi.resolution Module#
This script illustrates the functionality of the trinidi.resolution
submodule.
[2]:
import numpy as np
import matplotlib.pyplot as plt
from trinidi import cross_section, resolution, util
Overview#
The resolution operator, \(R\), models the uncertainty distribution of the emission time of the neutrons. In the idealized case, when the neutron pulse is instantaneous, the time-of-arrival (TOA) is equal to the time-of-flight (TOF) and the resolution operator is equal to the identity: \(R = I\). However, in practice the neutron pulse often has a significant durantion and match the TOA (i.e. the measured time at the detector plane) does not deterministically the TOF (i.e. the neutron energy).
If \(Z\) is the array of areal densities and \(D\) is the cross section dictionary, then the expected transmission \(Q\) is
In this script we will generate artificial raw transmission data, \(Q_0\), and the resolution operator \(R\) to generate the blurred transmission spectrum \(Q = Q_0 R\). We define the blurred transmission spectrum to correspond to the TOA sampling vector \(t_A\) and the raw transmission to correspond the the TOF sampling vector, \(t_F\). The \(t_A\) samples are a subset of the \(t_F\) samples so that boundary effects due to the blurring are avoided.
Defining the TOA sampling vector#
By our convention, the \(t_A\) vector has equidistant and increasing sampling.
[3]:
Δt = 0.30 # bin size in μs
t_A = np.arange(72, 450, Δt)
N_A = t_A.size # number of TOA bins
The corresponding energy range of the neutrons is computed and displayed below. Note that the corresponding energy samples are neither equidistant nor increasing.
[4]:
flight_path_length = 10 # flight path length in m
E = util.time2energy(t_A, flight_path_length)
print(f"t_A = [{t_A[0]:.3f}, {t_A[1]:.3f}, ..., {t_A[-1]:.3f}] μs")
print(f"E = [{E[-1]:.3f}, {E[-2]:.3f}, ..., {E[0]:.3f}] eV")
t_A = [72.000, 72.300, ..., 449.700] μs
E = [2.585, 2.588, ..., 100.830] eV
Defining the Output Shape#
When defining the resolution operator, the output_shape
of the operator must be passed to the constructor. The output_shape
is equal to projection_shape + (N_A,)
where projection_shape
is usually the size of the detector.
[5]:
projection_shape = (3, 3)
output_shape = projection_shape + (N_A,)
Defining a Resolution Operator#
In this example we use a set of primitive rectangular convolution kernels. The t_F
TOF sampling vector is retrieved from R
and in general it is larger than t_A
.
[6]:
kernels = [np.ones(10) / 10, np.ones(20) / 20, np.ones(30) / 30]
R = resolution.ResolutionOperator(output_shape, t_A, kernels=kernels)
t_F = R.t_F # the TOF sampling vector
N_F = t_F.size
print(f"t_A = [{t_A[0]:.3f}, {t_A[1]:.3f}, ..., {t_A[-1]:.3f}] μs")
print(f"t_F = [{t_F[0]:.3f}, {t_F[1]:.3f}, ..., {t_F[-1]:.3f}] μs")
print(f"{N_F = } ≥ {N_A = }")
t_A = [72.000, 72.300, ..., 449.700] μs
t_F = [69.450, 69.750, ..., 454.050] μs
N_F = 1283 ≥ N_A = 1260
We can display the resolution operator attributes like this:
[7]:
print(R)
<class 'trinidi.resolution.ResolutionOperator'>
input_shape = (3, 3, 1283) = projection_shape + (N_F,)
output_shape = (3, 3, 1260) = projection_shape + (N_A,)
projection_shape = (3, 3)
N_F = 1283
N_A = 1260
Next, we can apply the resolution operator to a synthetic transmission spectrum.
Below we define a cross section dictionary, D
, using the t_F
sampling vector. The areal densities, Z
are equal to z.T
at every pixel.
[8]:
isotopes = ["Pu-239", "U-235"]
N_M = len(isotopes) # number of isotopes
z = np.array([[1e-3], [2e-3]]) # areal density in mol/cm²
Z = np.ones(projection_shape + (1,)) @ z.T # 2D areal density map
print(f"{Z[0,0] = } [mol/cm²]") # printing first pixel areal densities
D = cross_section.XSDict(isotopes, t_F, flight_path_length) # cross section in cm²/mol
Z[0,0] = array([0.001, 0.002]) [mol/cm²]
The synthetic raw spectrum, Q0
, and the blurred spectrum Q
are computed and plotted below. We also plot the associated weights that indicate at which TOF which kernels are active.
[9]:
Q0 = np.exp(-Z @ D.values)
Q = R(Q0)
fig, ax = plt.subplots(2, 1, figsize=[8, 4], sharex=True)
ax = np.atleast_1d(ax)
ax[0].plot(t_F, Q0[0, 0], label="Q0", alpha=0.75)
ax[0].plot(t_A, Q[0, 0], label="Q", alpha=0.75)
ax[0].legend(prop={"size": 8})
ax[0].set_title("Raw vs. Blurred Transmission Spectrum")
R.plot_kernel_weights(ax[1])
plt.show()
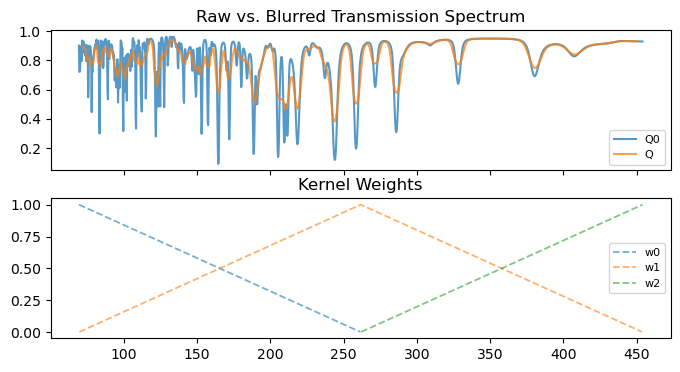
Defining a General Resolution Operator#
If we have a function that computes the blurring kernel as a function of TOA, below we show how we can create the corresponding kernels. We illustrate this using the built-in lansce_fp5_kernel
function and the equispaced_kernels
function.
The lansce_fp5_kernel
function computes the kernel shape as a function of TOA and other parameters for a particular flight path facility. Below we generate the kernel at the center TOA.
[10]:
i = N_A // 2 # center index
kernel = resolution.lansce_fp5_kernel(t_A[i], Δt, flight_path_length)
fig, ax = plt.subplots(1, 1, figsize=[12, 8], sharex=True)
ax = np.atleast_1d(ax)
ax[0].plot(kernel, label="kernel", alpha=0.75)
ax[0].legend(prop={"size": 8})
fig.suptitle(f"Single lansce_fp5_kernel at t_A = {t_A[i]:.2f} μs")
# plt.savefig('')
plt.show()
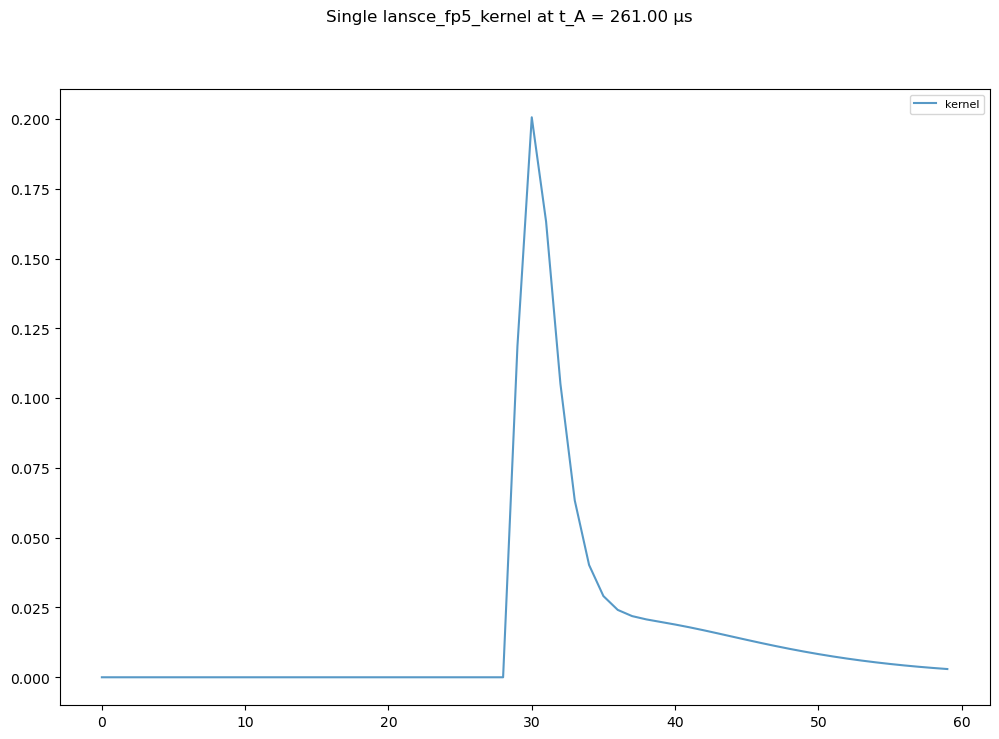
For the resolution operator we require these kernels at equispaced TOAs. First we need a function g
with a single argument, t_A
.
[11]:
# i.e. g(t_A) == resolution.lansce_fp5_kernel(t_A, Δt, flight_path_length)
g = lambda t_A: resolution.lansce_fp5_kernel(t_A, Δt, flight_path_length)
The equispaced_kernels
function generates the appropriate kernels.
[12]:
num_kernels = 5
kernels, t_As = resolution.equispaced_kernels(t_A, num_kernels, g)
fig, ax = plt.subplots(1, 1, figsize=[8, 3], sharex=True)
ax = np.atleast_1d(ax)
for i, kernel in enumerate(kernels):
ax[0].plot(kernel, label=f"kernel[{i}] at t_A={t_As[i]:.2f} μs", alpha=0.75)
ax[0].legend(prop={"size": 8})
fig.suptitle(f"lansce_fp5_kernel kernels")
# plt.savefig('')
plt.show()
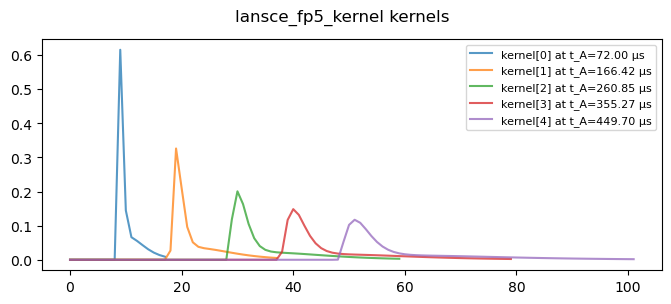
Below we show the resulting synthetic spectra.
[13]:
R = resolution.ResolutionOperator(output_shape, t_A, kernels=kernels)
t_F = R.t_F
D = cross_section.XSDict(isotopes, t_F, flight_path_length) # cross section in cm²/mol
Q0 = np.exp(-Z @ D.values)
Q = R(Q0)
fig, ax = plt.subplots(2, 1, figsize=[8, 4], sharex=True)
ax = np.atleast_1d(ax)
ax[0].plot(t_F, Q0[0, 0], label="Q0", alpha=0.75)
ax[0].plot(t_A, Q[0, 0], label="Q", alpha=0.75)
ax[0].legend(prop={"size": 8})
ax[0].set_title("Raw vs. Blurred Transmission Spectrum")
R.plot_kernel_weights(ax[1])
plt.show()
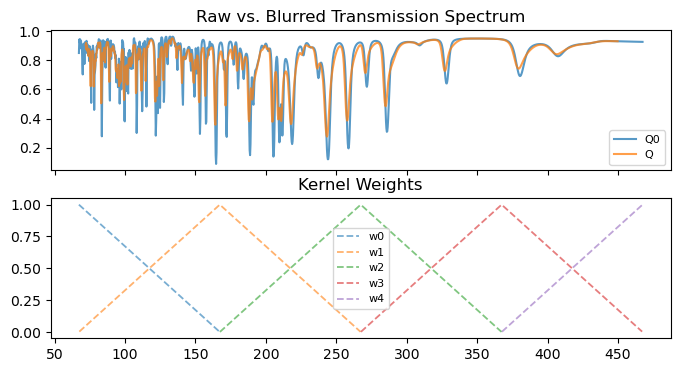
Identity Resolution Operator#
If it is desired to omit the resolution operator, you can simply create an identity resolution operator setting the kernels=None
argument.
[14]:
R = resolution.ResolutionOperator(output_shape, t_A, kernels=None)
t_F = R.t_F
N_F = t_F.size
Note: This is equivalent to using a single dirac kernel:
[15]:
print(R.kernels)
[array([1])]
Only in the case of the identity operator the TOF and TOA sampling vectors are equal to each other and have the same length, i.e. R is a square linear operator.
[16]:
if np.allclose(t_F, t_A) and (N_F == N_A):
print("t_F == t_A")
print(f"N_F == N_A == {N_A}")
t_F == t_A
N_F == N_A == 1260
Below we show the resulting synthetic spectra (which are of course equal).
[17]:
D = cross_section.XSDict(isotopes, t_F, flight_path_length) # cross section in cm²/mol
Q0 = np.exp(-Z @ D.values)
Q = R(Q0)
fig, ax = plt.subplots(2, 1, figsize=[8, 4], sharex=True)
ax = np.atleast_1d(ax)
ax[0].plot(t_F, Q0[0, 0], label="Q0", alpha=0.75)
ax[0].plot(t_A, Q[0, 0], label="Q", alpha=0.75)
ax[0].legend(prop={"size": 8})
ax[0].set_title("Raw vs. Blurred Transmission Spectrum")
R.plot_kernel_weights(ax[1])
plt.show()
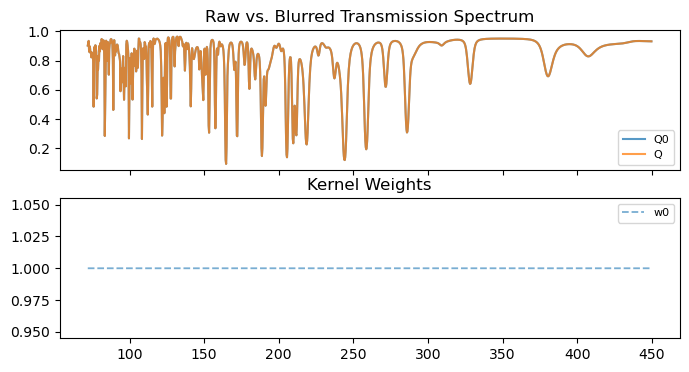